Non-homogeneous random walks
One of the themes of some of my research over the last few years has been on spatially non-homogeneous random walks; this has included joint work with Nicholas Georgiou, Ostap Hryniv, Chak Hei Lo, Iain MacPhee, Mikhail Menshikov, Aleksandar Mijatović, and Serguei Popov. With Menshikov and Popov we wrote a book covering some of this.
A $d$-dimensional random walk is, in this context, a discrete-time time-homogeneous Markov process on $d$-dimensional Euclidean space. If the random walk is spatially homogeneous, its increments are independent and identically distributed. These classical random walks still generate interesting problems, but it is of interest to study non-homogeneous walks, where the increment distribution varies from place to place in some systematic way.
Lamperti's problem
In the case of one dimension, important early work was done by J. Lamperti. He showed that the asymptotic behvaiour of the process is governed primarily by the mean and variance of the increments (as a function of the current position), at least in the case where the increment variance is uniformly bounded. Such Lamperti processes include discrete analogues of the Bessel process, and are prototypical near-critical stochastic systems.
Click on the buttons below for some of my work related to this class of processes.
Anomalous recurrence
In one dimension, a zero drift homogeneous random walk is recurrent. For a non-homogeneous random walk, zero drift is not by itself enough to guarantee recurrence (although it does ensure that the walk changes sign infinitely often, so counterexamples have infinite variance). Even in the case of bounded increments, however, zero drift walks in higher dimensions can exhibit very different behaviour from what one would expect from the homogeneous case.
This anomalous recurrence behaviour means that there exist zero-drift random walks in two dimensions that are transient, and zero-drift random walks in three (or higher) dimensions that are recurrent. Such examples can be achieved by letting the increment covariance matrix vary in a particular way throughout space. See the pictures below. For a canonical family of examples, we show weak convergence of the random walk to a limiting diffusion. The class of limiting diffusions has a skew-product structure much richer than that displayed by ordinary Brownian motion, since these diffusions can be recurrent in higher dimensions.
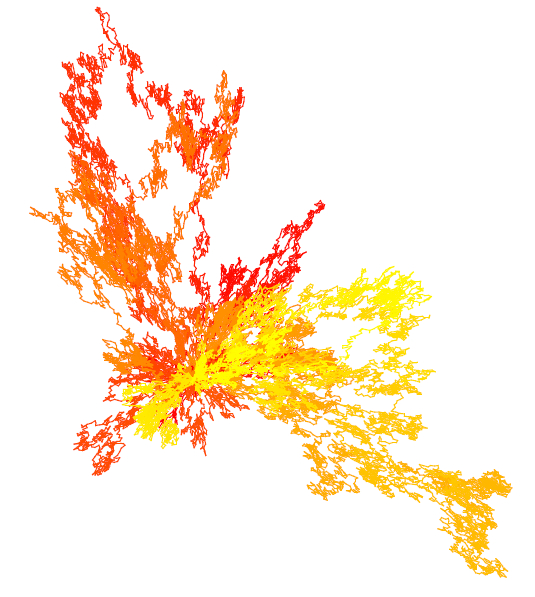
Simulation of a zero-drift random walk that is more recurrent than ordinary planar random walk. This is achieved by varying the increment covariance matrix so that the ellipse of the corresponding quadratic form is elongated in the radial direction.
Image by Nicholas Georgiou
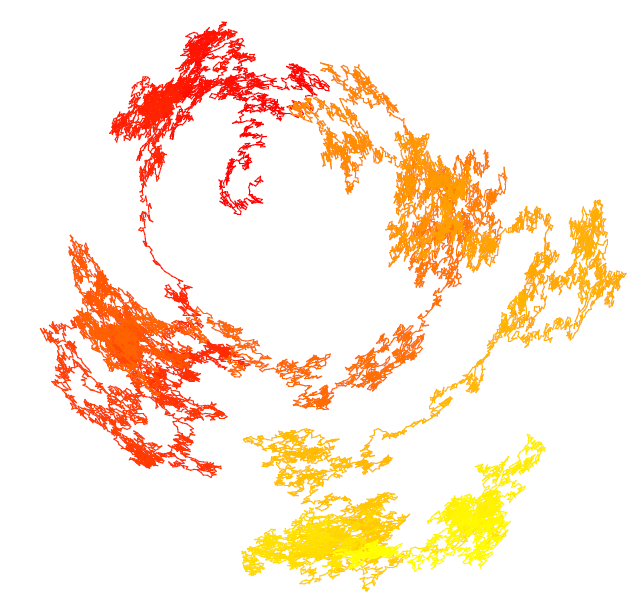
Here is a simulation of a zero-drift random walk that is transient in the plane. This time the ellipse is elongated in the transverse direction.
Image by Nicholas Georgiou
Multidimensional walks with asymptotically zero drift
The classical situation can be retrieved if one assumes certain regularity conditions, including a fixed increment covariance structure, ruling out the anomalous behviour described above. In that setting, to probe the recurrence-transience phase transition one is led to investigate non-homogeneous random walks with asymptotically zero mean-drift; for such walks, the magnitude of the drift at a point tends to 0 as the distance of that point from the origin tends to infinity. This is the higher-dimensional analogue of Lamperti's problem in one dimensions.
These models are sometimes known as centrally biased random walks.